Evaluating AI solutions for patent management – a tactical checklist
AI is transforming patent management, but how do you ensure a tool delivers on its promises? In this blog post, we recap the insights from Andreas Cehlinder’s recent webinar on evaluating AI tools for IP workflows.
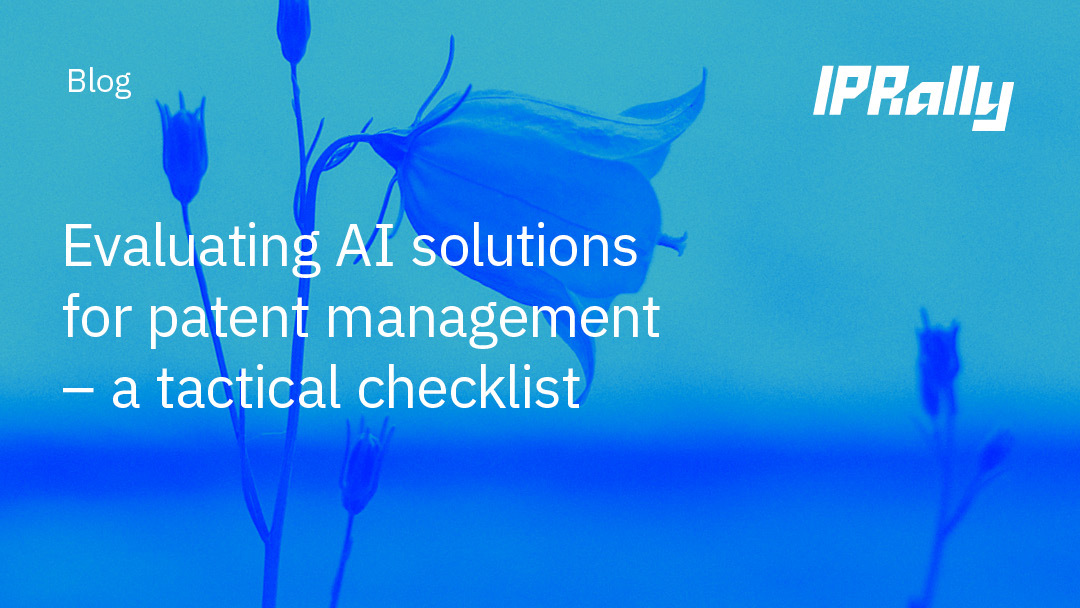
We're moving beyond the initial AI hype into an era of practical implementation, where AI is becoming an integral part of patent professionals' workflows. The ability to efficiently search, analyze, and act on patent information has never been more critical. But how can you be sure that the AI tools you're considering will truly meet their promises and add value?
Recently, our Chief Commercial Officer, Andreas Cehlinder, explored this topic in a webinar hosted in collaboration with In-House Connect. In this blog post, we summarize the key insights and takeaways from the session to help you navigate the evolving landscape of AI-powered patent management.
A tactical checklist for evaluating AI solutions
A practical, data-driven approach is crucial when evaluating AI tools for patent management. A well-structured tactical checklist helps professionals identify the right solution while ensuring it meets key criteria like quality, security, and ROI expectations.
However, this checklist only scratches the surface. We are currently working on a more comprehensive guide that will dive deeper into the evaluation process and provide further insights, which we look forward to sharing soon.
1. Quality and capabilities
A good practice is to start evaluations with simple eye tests, gradually increasing the scientific rigor toward more objective metrics like precision and recall. If the AI doesn’t initially appear to meet your needs, it’s crucial to investigate why and identify potential adjustments.
Some AI tools, particularly those relying on large language models, can deliver confident yet inaccurate results, highlighting the need for systematic and robust testing.
- Perform an eye test: Run a quick screening to assess the perceived subjective quality of the results and see if the AI delivers within expectations. Most products pass this test, but if not, a few test runs might be enough to weed out obviously immature or poorly trained solutions.
- Conduct real-life tests: Taking the eye test a step further, run a few actual cases through the AI tool to assess its real-world performance. This will help determine if it can address the problem more effectively than your traditional tools. Use these real cases to evaluate whether the tool reduces effort, accelerates processes, and delivers more accurate or comprehensive results.
- Regression tests: Compare historical data, such as search reports, patent drafts, or third-party analyses, with AI-generated outputs. This method is useful for validating accuracy and ensuring the AI performs at least as well as your current tools. Are the results the same, better or worse? Was anything missed or added?
- Seek objective benchmarks: Standardized metrics, like precision and recall, are very effective for evaluating competing solutions. Sometimes there are also golden standard datasets you can ask the providers to run. Unfortunately, there are very few universal benchmarks for accuracy, or gold data sets, in the patent applications space, but there are a few. Whatever you use, make sure to use the same golden questions or data sets when you compare solutions. Above all, always demand transparency from your providers to ensure a fair and thorough evaluation.
2. Return on investment (ROI)
AI solutions can deliver impressive ROI, but it’s important to go beyond initial costs to evaluate the long-term value. By using AI, organizations can significantly reduce the time spent on repetitive tasks like patent searches and drafting. In addition, replacing outdated systems or outsourcing services can result in substantial cost savings.
Risk mitigation is also an important aspect here, with the potential to reduce patent litigation risks or improve patent grant ratios potentially providing significant returns. AI also opens up new opportunities to conduct more strategic analyses that were previously unattainable. On average, the ROI from adopting AI in legal is about 350% in the first 14 months.
- Quantify time savings: Measure how much faster tasks can be completed using AI and the monetary value of each time unit. This is relatively straightforward if you can track time in both the new and old methods.
- Assess cost reductions: Identify outdated tools or outsourced services that could be replaced by the AI solution. Consider both tangible and intangible cost savings, such as reduced filing expenses resulting from a higher grant rate or fewer office actions. Evaluate how the AI tool might streamline processes and eliminate inefficiencies, ultimately leading to cost savings across the board.
- Evaluate risk mitigation: Estimate the financial impact of reducing risks, such as patent infringement or litigation.
- Explore new opportunities: Consider how AI might enable processes or insights that were previously out of reach.
3. Information and security
No matter how impressive an AI solution's performance may be, weak security measures are typically a deal breaker in the patent domain. Demand transparency from your providers to ensure a fair and thorough evaluation of everything from data encryption to data ownership and regulation compliances. At IPRally, we address this need through our comprehensive Trust Center, providing clear and accessible information on our security practices. Below, we present checklists for three main domains in security assessment to guide your evaluation.
Data and information security
Ensuring robust data security is critical when evaluating AI solutions. From encryption protocols to access control and incident response plans, every layer of protection matters. This should be part of the evaluation, not an afterthought.
- What kind of data encryption and protection methods do the providers use? Does this meet your requirements for data security
- What type of access control do the providers offer, and are their policies compliant with your internal requirements?
- What is the providers’ incident response protocol? Is it satisfactory?
- What security protocols and technology do the used cloud providers have
- in place? Reputable providers? Does infrastructure, location and policies matter?
- Do providers have security certifications (ISO, SOC etc), and is this required?
Compliance and legal
You will likely be sharing both sensitive and generic data with the AI provider, and you need to ensure that both you and they are compliant with any and all regulations, both internal and external.
- What local, federal and international regulations for data processing are relevant, and is the provider fully compliant with e.g. privacy laws for personal Identifiable data (PII) and for unidentifiable, aggregate or derivative data?
- Will any type of restricted data types be processed by the provider? What processes and limitations are required for national interest information, export-controlled and for medical data, government-funded research etc?
- Does the provider comply with any additional internal regulations for data processing?
AI Model training, integrity and ownership
Model training, integrity and ownership are usually not regulated in laws and regulations, unless internal. But, these are some of the most important, and highest hurdles to address, especially with Generative AI.
- Who owns uploaded, generated and derivative data used in the Ai, and how can this be used?
- Pre-existing?
- User-generated
- AI-generated (derivative)
- Is data and behavior used to train models (and are these general or proprietary)? If so, what type of data, and what type of models?
- What type of security is in place to safeguard the actual AI models (model security)?
- What about ISO 42001 for AI systems?
4. Adoption
Even the best AI solutions will fall short if they are not adopted effectively. A successful AI implementation relies heavily on how well the tool integrates into the team's workflow. A key challenge is overcoming the adoption barrier – getting the team comfortable with using AI on a daily basis.
AI tools should be user-friendly, with intuitive UI/UX design that makes it easy for users at all levels to get up to speed. Strong training and support systems can make a significant difference in user adoption. As users begin to use AI tools, it’s vital to ensure they feel confident and capable of using them to their full potential.
Demographics and use cases
Considerations about who will be using the AI, and for what, are a lot rigid, but still impactful when assessing AI solutions for wider implementation. If no one uses the tools, the tools are useless.
- Which demographics need to be part of the rollout for the implementation to have maximum effect?
- What are each user group expected to do?
- How do you, and the provider, need to win them over and enable them?
(in)Experience and capabilities
Mapping out, and confirming, the maturity of the potential users is important as resistance and skill gaps could be one of the biggest barriers to adoption.
- How much does each user, or user group, understand about the process?
- How much does each user, or user group, understand about AI?
- What is each user group’s experience with performing the tasks?
UI and UX
In addition to assessing the results, quality and security, the usability of the solutions should also be part of your assessment.
- Is the tool well-designed and easy to use for each of the different user groups?
- Will you require a lot of resources to train the users, or is the tool intuitiveand adaptable to your requirements?
- Is there a way to provide feedback once implemented?
- What type of assistance do you need from the provider? Are there costs associated with this? Is the provider mature enough to provide support?
5. In summary
The challenges in the patent domain are immense, but AI holds the potential to transform the way IP professionals work. A thorough evaluation process, guided by a comprehensive checklist, is key to finding the right solution. Focus on aligning technology with your team’s needs, ensuring security, and preparing for future advancements.
Innovation may be driven by technology, but change is driven by people. With the right tools, processes, and mindset, AI can revolutionize your approach to patent management – while saving time, reducing costs, and uncovering new opportunities.
Ready to explore one of the leading tools?
There’s no better way to understand the potential of AI in patent management than by experiencing it firsthand. That’s why we’re inviting you to try IPRally for free. Sign up for your free trial today and take the first step toward smarter, faster patent management.