A leap forward in IPRally's accuracy, efficiency and scalability
We're excited to introduce two new updates that push the boundaries of AI-driven patent search even further. Our latest advancements – an enhanced text-to-graph model and a powerful new node reranker – are designed to make your search experience more accurate and efficient. With the addition of the reranker, we now apply a second layer of ranking to search results, improving the quality of the top results. The improved graph parser not only boosts search accuracy but also lays the foundation for significant future developments. Here’s a look at what’s new and, more importantly, how it benefits you.
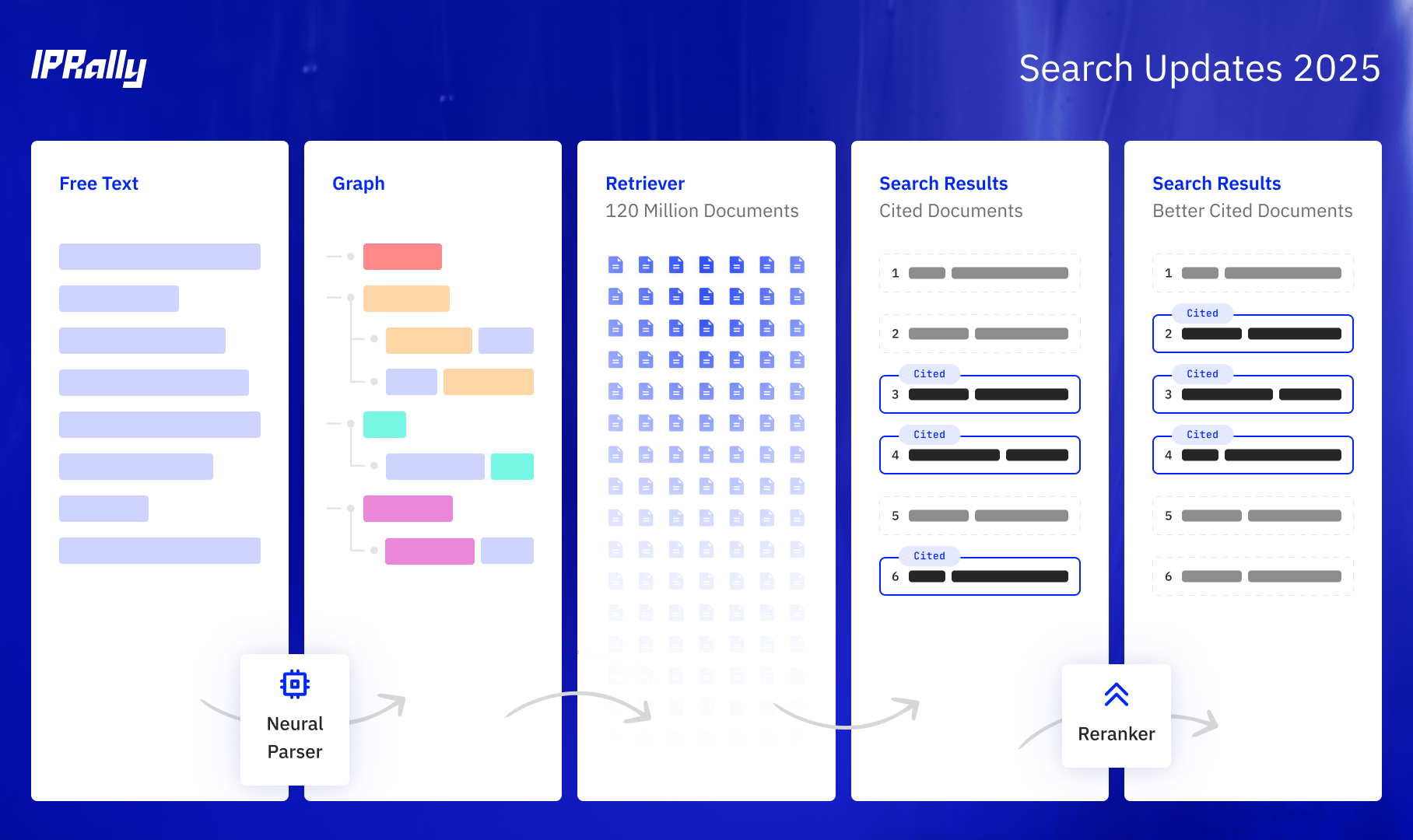
Next-generation graph parsing: precise and scalable
One of IPRally's core strengths is transforming free-text inputs into structured graphs, which serve as search queries for our AI-powered patent search. Recognizing the long-term scalability limitations of our previous graph parser, we have developed a more scalable and accurate model, incorporating the latest advancements in deep learning and natural language processing.
What’s changing?
The previous method relied on a set of heuristics and algorithms to convert free text into graph-based search queries. Now, we’re introducing a single neural network built on a pre-trained language model. This shift not only enhances the accuracy of graph parsing but also provides a much stronger foundation for continuous improvements going forward.
Performance improvements
- More accurate search queries: The new model better interprets free-text inputs, ensuring that the generated graphs more accurately represent your intended search.
- Faster performance: The graph parsing service is now at least 3–5 times faster, particularly benefiting searches with long inputs (e.g., claims in chemical patents).
- Improved search results: With better graphs, the recall increases and your search queries yield more relevant results, helping you find prior art more efficiently.
While the UI remains unchanged, you may notice subtle differences in the generated graphs and a slight improvement in the relevance of search results. The most significant enhancements related to the new graph parser, however, are yet to come.
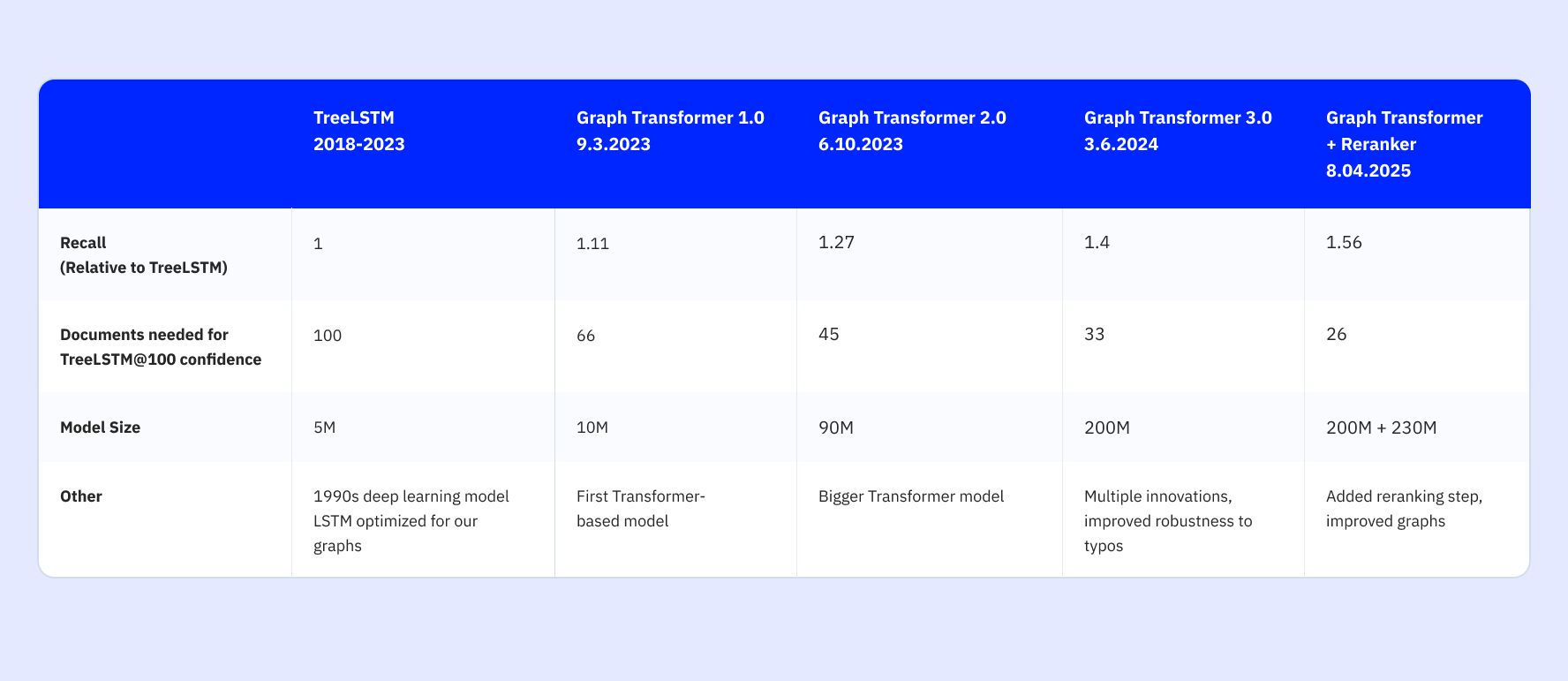
A second layer of relevance: Introducing the node reranker
Patent search isn’t just about retrieving documents – it’s about ranking them in a way that puts the most relevant results at the top. Our new node reranker enhances this process by refining the way search results are ordered, ensuring that the most relevant patents surface first.
How does it work?
IPRally’s AI search already uses graphs, where patents are broken down into nodes (representing different parts of an invention) and edges (showing relationships between the parts). The node reranker works by:
- Comparing at the node level: Instead of treating patents as a whole, the model compares individual nodes from your search query with nodes from the search results.
- Re-ranking the search results: After retrieving the search results, the reranker evaluates them once again based on this granular comparison, ensuring the best matches rise to the top.
Performance improvements
- More relevant results on top: By re-ranking search results at a more detailed level, you’ll spend less time sifting through less relevant patents.
- Improved recall: Our internal tests show an 11.33% improvement in recall for the top 5 search results when reranked from the top 200 production search results. This translates to roughly a 20% reduction in the number of results you need to review to find the same relevant documents.
Toward full automation
Both of these updates reinforce IPRally’s position as a leader in AI-powered patent search. With a more intelligent approach to graph parsing and a powerful new ranking mechanism, users will benefit from more precise searches and highly relevant search results at the top.
We’ll continue to push forward with the automation of repetitive, substance-level patent work, doing so in a transparent, verifiable, and trustworthy manner. You can read more about our vision in this blog post by our CPO and Co-founder, Sakari Arvela.
Stay tuned for more improvements, and as always, we’d love to hear your feedback!